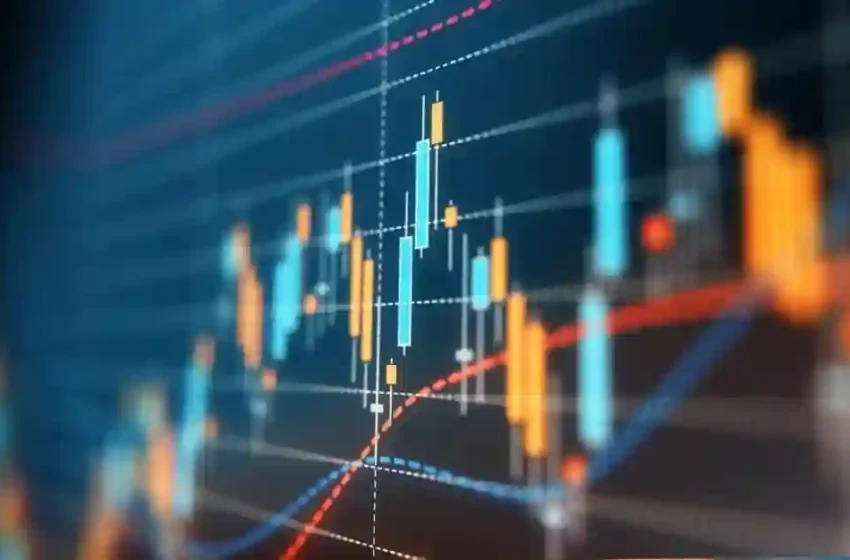
Analyzing Market Sentiment with Sentiment Analysis Forex Robots
In the fast-paced world of Forex trading, deciphering market sentiment is a pivotal factor influencing trading decisions and outcomes. Market sentiment, often driven by a complex interplay of economic indicators, geopolitical events, and investor psychology, plays a crucial role in shaping price movements and market trends. In recent years, the advent of sentiment analysis Forex robot has revolutionized the way traders interpret and capitalize on market sentiment. This article delves into the mechanics of sentiment analysis in Forex trading, exploring how sentiment analysis Forex robots leverage advanced algorithms to decode market sentiment and empower traders with actionable insights.
Understanding Market Sentiment:
Market sentiment refers to the overall attitude or emotional tone of market participants towards a particular asset, currency pair, or financial market. It encompasses a range of sentiments, including optimism, pessimism, confidence, fear, and greed, which influence trading behavior and drive price fluctuations. Market sentiment is often reflected in various indicators, such as investor surveys, news sentiment, social media chatter, and price action patterns, providing valuable cues for traders seeking to gauge market direction and sentiment trends.
The Role of Sentiment Analysis in Forex Trading:
Sentiment analysis, also known as opinion mining, is a data-driven approach to analyzing and quantifying market sentiment using natural language processing (NLP) techniques, machine learning algorithms, and computational linguistics. In the context of Forex trading, sentiment analysis involves extracting, processing, and interpreting textual data from news articles, social media posts, economic reports, and other sources to assess market sentiment and sentiment trends.
Sentiment analysis Forex robots are automated trading systems that leverage sentiment analysis algorithms to analyze textual data and derive sentiment scores or indicators. These robots employ a combination of techniques, including sentiment lexicons, machine learning classifiers, and neural networks, to classify and quantify the sentiment expressed in textual data. By analyzing vast amounts of textual data in real-time, sentiment analysis Forex robots provide traders with valuable insights into market sentiment dynamics and sentiment-driven price movements.
Key Components of Sentiment Analysis Forex Robots:
Data Collection and Aggregation:
Sentiment analysis Forex robots collect and aggregate textual data from a diverse range of sources, including financial news websites, social media platforms, economic calendars, and press releases. These robots utilize web scraping techniques, application programming interfaces (APIs), and data feeds to gather real-time textual data from relevant sources.
Natural Language Processing (NLP):
Natural language processing is a core component of sentiment analysis Forex robots, enabling them to understand, process, and analyze textual data in human language. NLP techniques, such as tokenization, part-of-speech tagging, syntactic parsing, and sentiment analysis, are employed to extract relevant information and sentiment indicators from textual data.
Sentiment Analysis Algorithms:
Sentiment analysis Forex robots utilize a variety of sentiment analysis algorithms to classify and quantify market sentiment expressed in textual data. These algorithms range from rule-based approaches and sentiment lexicons to supervised and unsupervised machine learning models. Sentiment lexicons, such as the Vader lexicon and the AFINN lexicon, assign sentiment scores to words or phrases based on their semantic meaning and context. Machine learning classifiers, including support vector machines (SVM), decision trees, and neural networks, learn to classify textual data into sentiment categories (e.g., positive, negative, neutral) based on labeled training data.
Sentiment Visualization and Reporting:
Sentiment analysis Forex robots provide traders with intuitive visualization tools and reports to visualize and interpret sentiment analysis results. These tools may include sentiment heatmaps, sentiment trend charts, sentiment distribution plots, and sentiment sentiment-based trading strategies.
Benefits of Sentiment Analysis Forex Robots:
Real-Time Insights:
Sentiment analysis Forex robots provide traders with real-time insights into market sentiment dynamics, enabling them to react quickly to sentiment-driven price movements and market trends.
Enhanced Decision-Making:
By incorporating sentiment analysis into their trading strategies, traders can make more informed and data-driven decisions, leveraging sentiment insights to anticipate market reversals, identify sentiment-driven trading opportunities, and manage risk effectively.
Improved Trading Performance:
Sentiment analysis Forex robots have the potential to improve trading performance and profitability by capturing sentiment-driven price movements and exploiting sentiment-based trading strategies.
Diversification of Trading Signals:
Sentiment analysis Forex robots complement traditional technical and fundamental analysis techniques, providing traders with additional trading signals and perspectives to enhance their trading strategies.
Challenges and Considerations:
While sentiment analysis Forex robots offer valuable insights into market sentiment dynamics, they also pose challenges and considerations for traders:
Data Quality and Accuracy:
The accuracy and reliability of sentiment analysis results depend on the quality and relevance of the textual data used for analysis. Traders must assess the credibility of data sources and account for potential biases or noise in sentiment analysis results.
Interpretation and Context:
Interpreting sentiment analysis results requires contextual understanding and domain expertise, as sentiment scores may vary depending on linguistic nuances, sarcasm, and cultural differences. Traders must exercise caution when interpreting sentiment analysis results and consider multiple factors influencing market sentiment.
Overfitting and Generalization:
Sentiment analysis models may suffer from overfitting or lack of generalization if trained on limited or biased datasets. Traders should evaluate the robustness and generalization capabilities of sentiment analysis models to ensure reliable performance across different market conditions.
Conclusion:
Sentiment analysis Forex robots represent a powerful tool for traders seeking to decode market sentiment and gain a competitive edge in Forex trading. By leveraging advanced algorithms and natural language processing techniques, these robots enable traders to analyze textual data, quantify market sentiment, and derive actionable insights to inform their trading decisions. While sentiment analysis Forex robots offer promising benefits for traders, they also pose challenges and considerations that require careful evaluation and management. As sentiment analysis continues to evolve, it is poised to become an indispensable component of traders’ toolkits, enhancing their ability to navigate the complexities of financial markets and capitalize on sentiment-driven trading opportunities.